#difference between data science and machine learning
Explore tagged Tumblr posts
Text
#difference between data science and machine learning#what is data science#data science#skills required for a data scientist
0 notes
Text
Complete Excel, AI and Data Science mega bundle.
Unlock Your Full Potential with Our 100-Hour Masterclass: The Ultimate Guide to Excel, Python, and AI.
Why Choose This Course? In todayâs competitive job market, mastering a range of technical skills is more important than ever. Our 100-hour comprehensive course is designed to equip you with in-demand capabilities in Excel, Python, and Artificial Intelligence (AI), providing you with the toolkit you need to excel in the digital age.
To read more click here <<
Become an Excel Pro Delve deep into the intricacies of Excel functions, formulae, and data visualization techniques. Whether youâre dealing with basic tasks or complex financial models, this course will make you an Excel wizard capable of tackling any challenge.
Automate Your Workflow with Python Scripting in Python doesnât just mean writing code; it means reclaiming your time. Automate everyday tasks, interact with software applications, and boost your productivity exponentially.
If you want to get full course click here <<
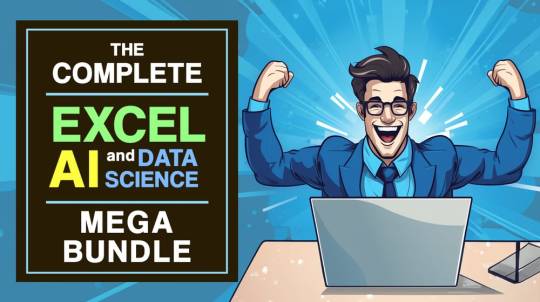
Turn Ideas into Apps Discover the potential of Amazon Honeycode to create custom apps tailored to your needs. Whether itâs for data management, content tracking, or inventory â transform your creative concepts into practical solutions.
Be Your Own Financial Analyst Unlock the financial functionalities of Excel to manage and analyze business data. Create Profit and Loss statements, balance sheets, and conduct forecasting with ease, equipping you to make data-driven decisions.
Embark on an AI Journey Step into the future with AI and machine learning. Learn to build advanced models, understand neural networks, and employ TensorFlow. Turn big data into actionable insights and predictive models.
Master Stock Prediction Gain an edge in the market by leveraging machine learning for stock prediction. Learn to spot trends, uncover hidden patterns, and make smarter investment decisions.
Who Is This Course For? Whether youâre a complete beginner or a seasoned professional looking to upskill, this course offers a broad and deep understanding of Excel, Python, and AI, preparing you for an ever-changing work environment.
Invest in Your Future This isnât just a course; itâs a game-changer for your career. Enroll now and set yourself on a path to technological mastery and unparalleled career growth.
Donât Wait, Transform Your Career Today! Click here to get full course <<
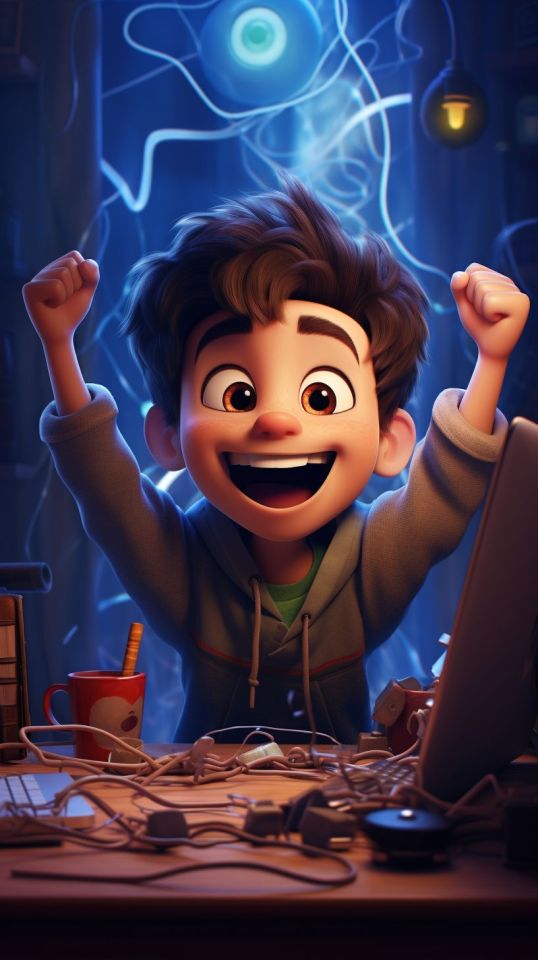
#data science#complete excel course#excel#data science and machine learning#microsoft excel#difference between ai and data science#learn excel#complete microsoft excel tutorial#difference between data science and data engineering#365 data science#aegis school of data science#advanced excel#excel tips and tricks#advanced excel full course#computer science#ms in data science#pgp in data science#python data science#python data science tutorial#Tumblr
1 note
¡
View note
Text
This is a good starting point but its not exhaustive by any means...
#Research 101: Part 1
##Â Â Â How to find a good research topic?
Itâs best to familiarize yourself with a discipline or topic as broadly as possible by looking beyond academia
Tips:
Be enthusiastic, but not unrealistic. For example, you might be tempted to throw yourself into finding out to what extent an entire economy has become circular, but it may already be challenging and tricky enough to find out which building materials are being recycled in the construction sector, and in what ways.
Be open-minded but beware of cul-de-sacs. You should always find out first whether enough is known about a topic already, or you might find yourself wasting a lot of time on it.
Be creative but stay close to the assignment. This starts with the topic itself; if one learning objective of the assignment is to carry out a survey, it isnât helpful to choose a topic for which you need to find respondents on the other side of the world. One place where you can look for inspiration is current events.Â
Although professors and lecturers tend to be extremely busy, they are often enthusiastic about motivated and smart students who are interested in their research field. You do need to approach them with focused questions, though, and not just general talk such as: âDo you know of a good topic for me?â In many cases, a good starting point is the scholar themselves. Do a search on them in a search engine, take a look at their university web page, read recent publications,
In most university towns, youâll come across organizations that hold regular lectures, debates, and thematic evenings, often in partnership with or organized by university lecturers and professors. If youâre interested in transdisciplinary research where academic knowledge and practical knowledge come together, this is certainly a useful place to start your search.
If you want to do interdisciplinary research, it is essential to understand and work with concepts and theories from different research fields, so that you are able to draw links between them (see Menken and Keestra (2016) on why theory is important for this). With an eye to your âinterdisciplinaryâ academic training, it is therefore a good idea to start your first steps in research with concepts and theories.
##How to do Lit Review:
Although texts in different academic disciplines can differ significantly in terms of structure, form, and length, almost all academic articles (research articles and literature reports) share a number of characteristics:
They are published in scholarly journals with expert editorial boards
These journals are peer-reviewed
These articles are written by authors who have no direct commercial or political interest in the topic on which they are writing
There are also non-academic research reports such as UN reports, data from statistics institutes, and government reports. Although these are not, strictly speaking, peer-reviewed, the reliability of these sources means that their contents can be assumed to be valid
You can usually include grey literature in your research bibliography, but if youâre not sure, you can ask your lecturer or supervisor whether the source youâve found meets the requirements.
Google and Wikipedia are unreliable: the former due to its commercial interests, the latter because anyone, in principle, can adjust the information and few checks are made on the content.
disciplinary and interdisciplinary search machines with extensive search functions for specialized databases, such as the Web of Science, Pubmed, Science Direct, and Scopus
Search methods All of these search engines allow you to search for scholarly sources in different ways. You can search by topic, author, year of publication, and journal name. Some tips for searching for literature: 1. Use a combination of search terms that accurately describes your topic. 2. You should use mainly English search terms, given that English is the main language of communication in academia. 3. Try multiple search terms to unearth the sources you need. a. Ensure that you know a number of synonyms for your main topic b. Use the search engineâs thesaurus function (if available) to map out related concepts.
During your search, it is advisable to keep track of the keywords and search combinations you use. This will allow you to check for blind spots in your search strategy, and you can get feedback on improving the search combinations. Some search engines automatically keep a record of this.
Exploratory reading How do you make a selection from the enormous number of articles that are often available on a topic? Keep the following four questions in mind, and use them to guide your literature review: â â What is already known about my topic and in which discipline is the topic discussed? â â Which theories and concepts are used and discussed within the scope of my topic, and how are they defined? â â How is my topic researched and what different research methods are there? â â Which questions remain unanswered and what has yet to be researched?
$$ Speed reading:
Run through the titles, abstracts, and keywords of the articles at the top of your list and work out which ideas (concepts) keep coming back.
Next, use the abstract to figure out what these concepts mean, and also try to see whether they are connected and whether this differs for each study.
If you are unable to work out what the concepts mean, based on the context, donât hesitate to use dictionaries or search engines.
Make a list of the concepts that occur most frequently in these texts and try to draw links between them.
A good way to do this is to use a concept map, which sets out the links between the concepts in a visual way.
All being well, by now you will have found a list of articles and used them to identify several concepts and theories. From these, try to select the theories and concepts that you want to explore further. Selecting at this stage will help you to frame and focus your research. The next step is to discover to what extent these articles deal with these concepts and theories in similar or different ways, and how combining these concepts and theories leads to different outcomes. In order to do this, you will need to read more thoroughly and make a detailed record of what youâve learned.
next: part 2
part 3
part 4
last part
#studyblr#women in stem#stem academia#study blog#study motivation#post grad life#grad student#graduate school#grad school#gradblr#postgraduate#programming#study space#studyspo#100 days of productivity#research#studyabroad#study tips#studying#realistic studyblr#study notes#study with me#studyblr community#university#student life#student#studyinspo#study inspiration#study aesthetic
44 notes
¡
View notes
Text
The Purpose of Language in General
I found that Language has three important uses is our every day life.
(1) The first one is obvious. Language as means of communication. We use language to communicate our ideas and desires to other people. duh!
(2) The second one is more subtle but also just as important. Language as means of articulating thought. We use language to construct and hold ideas together. Even if we do not like talking to people, language is incredibly important.
Ferrel children are children who grew in the wild with no access to human upbringing. These children are mentally handicapped and I believe this is largely due to lack of language. 10,000 years ago our brains where primitive but not very different from today. My personal hunch is that crude language skills leads to crude thinking. Thats how we got by in those days. And that is the difference between us modern humans and humans 10,000 years ago.
There are people who do not have an inner monologue yet they are very intelligent. I would bet that these people still have some sort of mechanism similar to language that allows them to articulate thought. Its based on other brain machinery such as vision and emotion.
(3) The third one was pointed out by a colleague. Language is a window to outside observation. We don't have to be involved in the conversation to understand what other people are talking about and learn from them. Documentation important also. History is preserved by written language. Only after written language did humanity started to thrive.
Sooo, what does language have to do with computer science, you may wonder. Well, programming languages are real languages. My claim is that programming is a communications problem. I see the machine as an alien species from ourselves. And the task at hand is to find a way to communicate to them and inform them what we want them to do.
(1) The first aspect (the obvious one) of programming languages is to do just that. We need to communicate to the machine what we want them to do.
(2) The second aspect (the more subtle one but just as important) of programming languages is for our sake to understand what we are telling the machine. We use programming languages to articulate ideas in our head before typing it on the computer. That is why we insist and persist on using JSON and XML to store data. Even thou those formats are incredibly inefficient from the view point of a machine.
(3) The third aspect of programming languages is a form of documentation. Other people can understand what the programmer is telling the machine. Its why open source is popular among programmers.
21 notes
¡
View notes
Note
Hiii! Sorry if I keep sending you more ask-
I was wondering what if Mayhem accidentally got transported into the TFP universe? (Considering it's the Idw comics, anything wacky is possible đ)
How will Soundwave from that universe react to Mayhem? And will Mayhem react to his 'dad'?
Thanks for the ask! Sorry for being so late.
In reality this could go down in many different ways.
TFP Soundwave, as far as I've seen him, holds Laserbeak as his own last shard of sanity, maybe in the past he had all of them and, well, the gladiator pits, the war, that little drone is all he has left, I don't know if in TFP the concept of sparkling or new sparks exist like it does on BV or this AU, but maybe Laserbeak was someone like that for him.
Having that in mind, as in by some fucked up chain of events (as in having Sunset near with his inherited bad and absurd luck), Mayhem were to fall in any other universe (because after the whole Brainstorm-made-a-time-machine-and-teared-the-whole-reality-apart well, now we have a multiverse!) you can bet that first and foremost Soundwave, as in the one in this reality, will tear even more the barriers between realities to have his sparkling back.
Meanwhile TFP Soundwave looks at the sparkling with a big interrogation mark on his visor, because where did he come from? How come his bio signature is so similar to his but he can't pinpoint from where, or what, the other part has come? How old is him? Depending of such, if Mayhem is still a newly forged mech then you can bet TFP Soundwave will guard him like a jealous mother (in that phase they aren't different from human babies), no one can look at such delicate little sparkle of joy, if Megatron asks nicely and is obviously in his right mind maybe he would consider it, if Mayhem was already an operative mech it would be so much difficult to hide him, and he has to drag him back more than once when he tries to reach the autobots, Mayhem has learned a lot from his sire back in his own reality, to this point everyone knows about him, the vehicons know better than stop him because he can only get so far before a pair of tentacles catch and drag him back again, no matter that his sharp digits claw at the ground, once back inside he is given a portion of energon to please be calm.
He would never let him interact with Starscream by obvious reasons, maybe Breakdown can talk more comfortable with him, but absolutely no permission to talk or interact or even be in the presence of Shockwave or Knockout, Soundwave doesn't like their look of "science" when they look at Mayhem.
Now with SG Soundwave, believe it or not, Mayhem feels even more horrified by different reasons, all his life his sire, his father, has always been taciturn, quiet, maybe a little gloomy, but overall he always thrived in his affection, while not so big like he has seen with other families, he knows his father loves him with little things.
SG Soundwave makes him sputter with his headband and overall colors, the way he talks and moves are so unnatural that make him feel itchy, but above all else what gets him totally out of his zone is that SG Soundwave makes a quick scanner at him, processes the data in two clicks, and soon tackles him down with a hug calling him "my baby".
In the SG universe they know of different realities thanks to Cliffjumper, and SG Soundwave has to recompose himself a moment and let go of the young mech, there is paint transfer in both of them due to the obvious crash.
If TFP Soundwave was creepy to Mayhem, he can't even start to describe SG Soundwave, but he does recognize him as creepy in his own way.
Obviously this is the most talkative Soundwave, "How many centuries are you? Have you been well from where you come from? Are you a medic? So proud! Do your do my young mech! Did you came from the hotspot in Kaon like me? If you didn't that's fine too!"
And obviously so, this Soundwave gets on the slightly different wavelength Mayhem does, "Do you have a sire? A carrier? Did I've you alone?" as he shows every other righteous decepticon the young mech, Mayhem has never seen other decepticons so fast and easy, they look happy to see him, totally different from his reality, "Such a handsome young lad!", somebot says, and SG Soundwave is quick to answer: "Sure is! Bet he got it from his other mentor!" as his visor shines green with absolute glee, the questions previously done return and Mayhem doesn't know if he should mangle this reality and tell him, he decided not to, because everything is backwards here, what if you are different too?
Mayhem doesn't want to answer, and he gets another cybertronian equivalent of aneurism when he meets the cassettes of this reality, at least he got to meet SG Ravage, because he never meet the one of his reality, never had the chance.
SG Soundwave is maybe the only one to help him return to his reality, retracting his battle mask to give a little peck on his helm, "Take care of you and the fam', Lil' doc".
Once Mayhem is safely returned, SG Soundwave makes a bee line to you, who seems to have a very bad day or your usual sour and tired expression is somehow worse, drinking some kind of human beverage to keep your sanity intact, but every ounce of sanity it's throw out of the window when he sits next to you, hands together as if he is begging or praying, your coffee is dripping from your mouth as he says "I wanna've your sparkling, my amor", because he recognized the wavelength of Mayhem's spark mimicking the one of a human, a human he knows very well.
Flatline has to come and help you as your coffee goes down the wrong way, Soundwave has this idea on his helm and nothing, nothing,will take it away.
Your destiny is sealed as Megatron looks at you helplessly, maybe you have a reason to date Soundwave now.
#transformers#x reader#tf mtmte#transformers x reader#reader insert#transformers idw#angst#transformers x human reader#tf soundwave#soundwave x human reader#soundwave x reader
67 notes
¡
View notes
Text
People often feel that their inner thoughts and feelings are much richer than what they are capable of expressing in real time. Entrepreneur Elon Musk is so bothered by what he calls this âbandwidth problem,â in fact, that one of his long-term goals is to create an interface that lets the human brain communicate directly with a compÂuter, unencumbered by the slow speed of speaking or writing.
If Musk succeeded, he would probably be disappointed. According to recent research published in Neuron, human beings remember, make decisions and imagine things at a fixed, excruciatingly slow speed of about 10 bits per second. In contrast, human sensory systems gather data at about one billion bits per second.
This biological paradox, highlighted in the new study, probably contributes to the false feeling that our mind can engage in seemingly infinite thoughts simultaneouslyâa phenomenon the researchers deem âthe Musk illusion.â Study co-author Markus Meister, a neuroscientist at the California Institute of Technology, says that âthe human brain is much less impressive than we might think. Itâs incredibly slow when it comes to making decisions, and itâs ridiculously slower than any of the devices we interact with.â
Meister and his co-author Jieyu Zheng, a doctoral candidate in neurobiology at Caltech, also highlight in their paper that our brain can do only one thingâslowlyâat a time. Even if Musk managed to hook his brain up to a computer, Meister says, he still wouldnât be able to communicate with it any faster than he could if he used a telephone.
The new research builds on decades of psychology studies showing that humans selectively perceive just a small portion of information from the sensory experience. âWe can only pay attention to so much, and thatâs what becomes our conscious experience and enters memory,â Meister says. What has been missing from past research, he continues, is âany sense of numbers.â He and Zheng have endeavored to fill that quantitative gap.
Meister and Zheng collated data from research across different fields, including psychology, neuroscience, technology and human performance. They used this informationâfrom the processing speed of single neurons to the cognitive prowess of memory championsâto run many of their own calculations so they could make comparisons between studies.
From research spanning nearly a century, they found that human cognition has repeatedly been measured as functioning at between about five and 20 bits per second, with a ballpark average of around 10 bits per second. âThis was a very surprising number,â Zheng says. Based on this finding, she adds, she and Meister also calculated that the total amount of information a person can learn across their lifetime could comfortably fit on a small thumb drive.
Human sensory systems such as sight, smell and sound, in contrast, operate much faster, the authors foundâat about 100,000,000 times the rate of cognition. âWhen you put these numbers together, you realize thereâs this huge gap,â Meister says. âFrom that paradox comes interesting new opportunities for science to organize research differently.â
The rich information relayed by our senses also contributes to a false notion that we register the massive amount of detail and contrast all around us. But thatâs âdemonstrably not true,â Meister says. When people are asked to describe what they see outside the center of their gaze, they âbarely make out anything,â he adds. Because our eyes have the capability to focus on any detail, he continues, âour mind gives us the illusion that these things are present simultaneously all the time,â even though in actuality we must focus on specific visual features to register them. A similar phenomenon occurs with mental ability. âIn principle, we could be having lots of different thoughts and direct our cognition in lots of different ways,â Meister says. âBut in practice, we can have only one thought at a time.â
Another problem that contributes to an overinflated sense of our own mind, he adds, is that we have no marker of comparison: âThereâs no way to step outside ourselves to recognize that this is really not much to brag about.â
The findings raise questions in many domains, from evolution and technology to cross-species comparisons, the authors write. One of the questions Meister and Zheng are most curious about, though, is why the prefrontal cortexâthought to be the seat of personality and behavioral controlâhouses billions of neurons yet has a fixed decision making capability that processes information at just 10 bits per second. The researchers suspect the answer might have something to do with the brainâs need to frequently switch tasks and integrate information across different circuits. But more complex behavioral studies will be needed to test that hypothesis.
Another important unanswered question, Meister says, is why the human brain can do only one thing at a time. âIf we could have 1,000 thoughts in parallel, each at 10 bits per second, the discrepancy wouldnât be as big as it is,â he says. Why humans are incapable of such mental multitasking is âa deep mystery that almost nothing is known about.â
Anthony Zador, a neuroscientist at Cold Spring Harbor Laboratory in New York State, who was not involved in the new paper but is mentioned in its acknowledgments, says the âwonderful and thought-Âprovokingâ study presents what seems to be a newly recognized fundamental truth about the brainâs upper limit of âroughly the pace of casual typing or conversation.â
âNature, it seems, has built a speed limit into our conscious thoughts, and no amount of neural engineering may be able to bypass it,â Zador says. âWhy? We really donât know, but itâs likely the result of our evolutionary history.â
Nicole Rust, a neuroscientist at the University of Pennsylvania, who also was not involved in the research, says the new study could reshape how neuroscientists approach some of their work.
âWhy can our peripheral nervous system process thousands of items in parallel, but we can do only one thing at a time?â she says. âAny theory of the brain that seeks to account for all the fascinating things we can do, like planning and problem-solving, will have to account for this paradox.â
15 notes
¡
View notes
Text
NP-Completeness
Machine learning #1
Complexity Recap
Assuming one already knows what algorithmic complexity is, I am writing this paragraph to explain it to those who don't. The complexity of an algorithm can be measured by its efficiency. So let's say I have a problem P for which I've proposed two algorithmsA and B as solutions. How could I determine which algorithm is the most efficient in terms of time and data support? Well, by using complexity ( space complexity and time complexity ). One way of measuring complexity is O notation.
 Exponential Complexity O(2^n)
In algorithms, this complexity arises when the time or space required for computation doubles with each additional element in the input. Problems with exponential complexity are typically NP-hard or NP-complete
P-Class and NP-Class
The P-class represents the set of polynomial problems, i.e., problems for which a solution can be found in polynomial time.
The NP-class represents the set of non-deterministic polynomial problems, i.e., problems for which a solution can be verified in polynomial time. This means it's easy to verify if a proposed solution is correct, but finding that solution might be difficult.
P = NP Question?
The question of whether P is different from NP is one of the most important problems in theoretical computer science. It is part of the seven problems selected by the Clay Institute in the year 2000, for which a reward of one million dollars is offered to whoever solves them.
NP-Complete Problem Class
NP-complete problems are problems that are both in the NP-class and are harder than all other problems in the NP-class.Solving any of these problems would be considered a major breakthrough in theoretical computer science and could have a significant impact in many areas such as optimization, planning, cryptography, etc. There is no specific monetary reward associated with solving an NP-complete problem, but it would be considered a significant achievement.
From an NP Problem to AnotherÂ
A polynomial reduction is a process that transforms an instance of one problem into an equivalent instance of another problem. In the case of polynomial reduction between NP-complete problems, this means that if you can efficiently solve problem B, then you can also efficiently solve problem A. This leads to the fact that if only one problem is solved from the list, then all the other problems will also be solved.
Approximation Algorithms
Computer scientists have devised approximation algorithms to efficiently solve NP-complete problems, even if finding an exact solution in polynomial time is not possible. These algorithms seek to find a solution that is close to optimality, although it may not be the optimal solution.
We can divide them into two categories:
Heuristics:Â These are explicit algorithms that propose solutions for NP-complete problems.
Metaheuristics:Â Unlike heuristics, metaheuristics are general strategies that guide the search for a solution without prescribing a specific algorithm.
#codeblr#code#css#html#javascript#java development company#python#studyblr#progblr#programming#comp sci#web design#web developers#web development#website design#webdev#website#tech#html css#learn to code
25 notes
¡
View notes
Text
lil personal rant. just need to voice my stressors to get them out. scroll if you'd like!
2 classes and one capstone project are all that stand between me and my Bachelors in Computer Science. It's been a long road, between starting college at 23 for some reason, switching majors after the loss of my grandfather, and so much in between . . . studying for these final two exams is pointless ( not really, but it feels pointless ) because everything I seem to read goes in one ear and out the other. I cannot retain anymore information. I study and tell myself that I'm almost there, but I just get exhausted sitting and staring at a computer or tablet screen, trying to ingrain these terms and concepts in my mind, not to mention doing countless computational problems so I have the formulas memorized for the exams. I have my capstone topic in mind ( a machine learning model that will analyze data collected from fine needle aspiration procedures performed on breast lesions, which will be trained to predict if a lesion is benign or malignant based on different features ) and have sent my proposal to my professor for approval. I'm great at getting these coding projects done. They're fun, almost like solving a puzzle, but I am so burnt out on tests and exams. I can't find a way to study that works. My program mentor called it senioritis, and maybe it is that, but I feel like I've hit a wall. If I don't study, I feel like a worthless human being. If I do study, I overdo it by studying for hours on end, exhaust myself, and just make myself feel even more depressed about everything than I already was.
I just want my degree so I can worry about finding a job. Did I mention every application I've sent since last year has been rejected? I got an email last night rejecting me due to too many applicants. They even said they wouldn't bother looking at my application. It's was awesome.
5 notes
¡
View notes
Text

In âThe Mercy of Gods,â the Authors of âThe Expanseâ Get Less Expansive
Like all good science fiction fans, Daniel Abraham enjoyed watching Andor. And Abraham knows what heâs talking about when it comes to high-quality sci-fi storytelling: Under the pseudonym James S.A. Corey, Abraham and Ty Franck cowrote the celebrated book series The Expanse and coproduced the television adaptation, which offered genre fans a captivating blend of space opera and political intrigue.
Nearly three years after The Expanse ended, both on-screen and on the page, the James S.A. Corey duo is out with the start of a new series: The Mercy of Gods, the first novel in a planned trilogy called The Captiveâs War. This book, which hit shelves last week, is both a satisfying stand-alone read and an excellent setup for the series to come, even if it forecasts a rather different sort of story from The Expanse.
Asked about a key distinction between the two series, Abraham turns to the gritty Star Wars prequel to a prequel to make a point.
Andor excelled because âit felt authentic,â Abraham says. âIt was the first time I can remember since the â70s when I felt like the Empire was really something oppressive, not just guys in cool, dark suits emoting a lot. It was this sense of the danger of that kind of vast machine.â
There is perhaps no machine more oppressive, more dangerous, and more vast than the Carryx empire in The Mercy of Gods: a race of warlike aliens who are set on conquering the galaxy and can slaughter millions without strain, due to their military might and incomprehensibly advanced technology. Andor is an apt comparison, as this new series details the flickers of a burgeoning rebellion against overwhelming imperial odds. âItâs survivors versus authoritarians,â Franck says. âIt is what happens to you when you are conquered by a militaristic authoritarian regime and you have to learn to live inside that regime.â
The Mercy of Gods starts on a human planetânot Earthâthousands of years in the future. Dafyd Alkhor is a research assistant in a biology lab, consumed by the petty desires and complications of any average human life: routine data collection, a workplace rivalry, a secret crush.
Then everything changes when the Carryx attack. They kill some humans and take others (including Dafyd) prisoner, transporting them back to their homeworld for a seemingly simple test: If the surviving humans can make themselves useful, theyâll live; if not, theyâll die, too.
The Captiveâs War employs a narrower narrative lens than The Expanse, at least in the first book. Almost all of the focus in Mercy is on Dafyd and his lab partnersâeach of whom develops as a unique and relatable character, just as James Holdenâs crew on the Rocinante flourish as both a collective unit and individual beings. But some of The Expanseâs other highlights, such as planet hopping and intricate politicking, are largely absent from the new novel.
This tightened focus extends to the seriesâ structure. While The Expanse spanned nine books, The Captiveâs War will be three. âItâs not doing the same kind of genre skipping that The Expanse did, because The Expanse did its Western, it did its noir, it did its political thriller,â Abraham says. âThe Captiveâs War is really, in a way, a more cohesive story than The Expanse had the ability to be.â
(Thatâs the high-minded way to look at the difference, at least. Franck offers a simpler explanation, with a laugh: âWe didnât want to write nine books again.â)
The new novel suffers from the lack of political maneuvering; thereâs no Chrisjen Avasarala or Winston Duarte analogue in Mercy. Its world can sometimes feel too small (though a development at the end of the book suggests an expansionâno pun intendedâto come in the sequel).
Yet at other points, Mercyâs world-building makes its universe feel unknowably gigantic. In The Expanse (spoiler alert), the alien threats never actually appear; the authors thought theyâd add more menace as looming, Lovecraftian specters. But in Mercy, aliens abound, as the human prisoners interact with and observe creatures of all shapes, sizes, and lifestyles.
Here, the authors utilize the delightful genre trick of implying a much larger world than is actually relevant to the plot. The human captives are housed in a massive pyramid where they encounter those multifarious species, but thatâs merely the prison for âthe other oxygen breathers,â Franck says. The humans also see other immense pyramids in the distance, which hold yet more aliens who live in sulfuric atmospheres, in water, and so on.
âThe idea of this is to give that sense of vast scale,â Abraham says. âThe idea is I want this to feel huge. I want this to feel complex.â
As is typical of a Corey novel, Mercy is also punctuated by moments of violence and humiliation and despair. The authors have always been able to turn darkness into page-turning thrills, and Mercyâs bleakest sections approachâif donât ever quite reachâthe worst protomolecule-induced horror that The Expanse ever presented.
But thereâs a light at the end of the tunnel. On the bookâs very first page, a flash-forward reveals that the Carryx empire falls and that Dafyd is somehow responsible. This choice was made partly for tonal balance, to compensate for all that darkness. âIf you didnât have some ray of hope, this would be a brutal read,â Abraham says.
Even more, it creates a compelling mystery that will carry through the rest of the series. The Carryx empire seems omnipotent and completely unbothered by humanity. It doesnât murder and enslave humans because of any hatred or rivalry; the humans are simply resources to be exploited. As one of the Carryx analogizes in the book, when a human cuts down a tree branch, âthe tree had no power to stop you, and so it became a tool in your hand.â
But somehow, the human tool named Dafyd will take down an empire. What could be an early spoiler is, instead, the engine for the rest of the plot. Franck explains, âWhen a guy says, âLet me tell you about the first time I killed a crocodile,â and then the scene opens with a guy being dropped naked into the middle of a crocodile pit, the question isnât, Did he survive and kill a crocodile? The question is, How the fuck did a naked guy in a crocodile pit actually beat one of them?â
That setup is reminiscent, incidentally, of Andor: Everyone watching Cassian, Luthen Rael, and Mon Mothma struggle against the might of the Empire knows that, eventually, the underdog rebels will succeed in creating the sunrise that Luthen knows heâll never see. But the tension and entertainment value come from learning how they reach that sunrise and how they endure all the dark nights they face along the way.Â
The same looks to be true of The Mercy of Gods. With all of its alien surroundings, and without Earth and our familiar solar system as a backdrop, this new series doesnât appear remotely as adaptable as The Expanse. It would be a surprise if Dafyd defeats the Carryx on television screens anytime soon.
But this story lives just as wonderfully on the page. âThe first book is telling you all the reasons why [the Carryx empire] canât fail: Itâs too big, itâs too powerful,â Franck says. âSo the tension is: What could this guy possibly have done to bring this about?â
5 notes
¡
View notes
Text
What are AI, AGI, and ASI? And the positive impact of AI
Understanding artificial intelligence (AI) involves more than just recognizing lines of code or scripts; it encompasses developing algorithms and models capable of learning from data and making predictions or decisions based on what theyâve learned. To truly grasp the distinctions between the different types of AI, we must look at their capabilities and potential impact on society.
To simplify, we can categorize these types of AI by assigning a power level from 1 to 3, with 1 being the least powerful and 3 being the most powerful. Letâs explore these categories:
1. Artificial Narrow Intelligence (ANI)
Also known as Narrow AI or Weak AI, ANI is the most common form of AI we encounter today. It is designed to perform a specific task or a narrow range of tasks. Examples include virtual assistants like Siri and Alexa, recommendation systems on Netflix, and image recognition software. ANI operates under a limited set of constraints and canât perform tasks outside its specific domain. Despite its limitations, ANI has proven to be incredibly useful in automating repetitive tasks, providing insights through data analysis, and enhancing user experiences across various applications.
2. Artificial General Intelligence (AGI)
Referred to as Strong AI, AGI represents the next level of AI development. Unlike ANI, AGI can understand, learn, and apply knowledge across a wide range of tasks, similar to human intelligence. It can reason, plan, solve problems, think abstractly, and learn from experiences. While AGI remains a theoretical concept as of now, achieving it would mean creating machines capable of performing any intellectual task that a human can. This breakthrough could revolutionize numerous fields, including healthcare, education, and science, by providing more adaptive and comprehensive solutions.
3. Artificial Super Intelligence (ASI)
ASI surpasses human intelligence and capabilities in all aspects. It represents a level of intelligence far beyond our current understanding, where machines could outthink, outperform, and outmaneuver humans. ASI could lead to unprecedented advancements in technology and society. However, it also raises significant ethical and safety concerns. Ensuring ASI is developed and used responsibly is crucial to preventing unintended consequences that could arise from such a powerful form of intelligence.
The Positive Impact of AI
When regulated and guided by ethical principles, AI has the potential to benefit humanity significantly. Here are a few ways AI can help us become better:
⢠Healthcare: AI can assist in diagnosing diseases, personalizing treatment plans, and even predicting health issues before they become severe. This can lead to improved patient outcomes and more efficient healthcare systems.
⢠Education: Personalized learning experiences powered by AI can cater to individual student needs, helping them learn at their own pace and in ways that suit their unique styles.
⢠Environment: AI can play a crucial role in monitoring and managing environmental changes, optimizing energy use, and developing sustainable practices to combat climate change.
⢠Economy: AI can drive innovation, create new industries, and enhance productivity by automating mundane tasks and providing data-driven insights for better decision-making.
In conclusion, while AI, AGI, and ASI represent different levels of technological advancement, their potential to transform our world is immense. By understanding their distinctions and ensuring proper regulation, we can harness the power of AI to create a brighter future for all.
8 notes
¡
View notes
Text
What's the difference between Machine Learning and AI?
Machine Learning and Artificial Intelligence (AI) are often used interchangeably, but they represent distinct concepts within the broader field of data science. Machine Learning refers to algorithms that enable systems to learn from data and make predictions or decisions based on that learning. It's a subset of AI, focusing on statistical techniques and models that allow computers to perform specific tasks without explicit programming.

On the other hand, AI encompasses a broader scope, aiming to simulate human intelligence in machines. It includes Machine Learning as well as other disciplines like natural language processing, computer vision, and robotics, all working towards creating intelligent systems capable of reasoning, problem-solving, and understanding context.
Understanding this distinction is crucial for anyone interested in leveraging data-driven technologies effectively. Whether you're exploring career opportunities, enhancing business strategies, or simply curious about the future of technology, diving deeper into these concepts can provide invaluable insights.
In conclusion, while Machine Learning focuses on algorithms that learn from data to make decisions, Artificial Intelligence encompasses a broader range of technologies aiming to replicate human intelligence. Understanding these distinctions is key to navigating the evolving landscape of data science and technology. For those eager to deepen their knowledge and stay ahead in this dynamic field, exploring further resources and insights on can provide valuable perspectives and opportunities for growthÂ
5 notes
¡
View notes
Text
The Mathematical Foundations of Machine Learning
In the world of artificial intelligence, machine learning is a crucial component that enables computers to learn from data and improve their performance over time. However, the math behind machine learning is often shrouded in mystery, even for those who work with it every day. Anil Ananthaswami, author of the book "Why Machines Learn," sheds light on the elegant mathematics that underlies modern AI, and his journey is a fascinating one.
Ananthaswami's interest in machine learning began when he started writing about it as a science journalist. His software engineering background sparked a desire to understand the technology from the ground up, leading him to teach himself coding and build simple machine learning systems. This exploration eventually led him to appreciate the mathematical principles that underlie modern AI. As Ananthaswami notes, "I was amazed by the beauty and elegance of the math behind machine learning."
Ananthaswami highlights the elegance of machine learning mathematics, which goes beyond the commonly known subfields of calculus, linear algebra, probability, and statistics. He points to specific theorems and proofs, such as the 1959 proof related to artificial neural networks, as examples of the beauty and elegance of machine learning mathematics. For instance, the concept of gradient descent, a fundamental algorithm used in machine learning, is a powerful example of how math can be used to optimize model parameters.
Ananthaswami emphasizes the need for a broader understanding of machine learning among non-experts, including science communicators, journalists, policymakers, and users of the technology. He believes that only when we understand the math behind machine learning can we critically evaluate its capabilities and limitations. This is crucial in today's world, where AI is increasingly being used in various applications, from healthcare to finance.
A deeper understanding of machine learning mathematics has significant implications for society. It can help us to evaluate AI systems more effectively, develop more transparent and explainable AI systems, and address AI bias and ensure fairness in decision-making. As Ananthaswami notes, "The math behind machine learning is not just a tool, but a way of thinking that can help us create more intelligent and more human-like machines."
The Elegant Math Behind Machine Learning (Machine Learning Street Talk, November 2024)
youtube
Matrices are used to organize and process complex data, such as images, text, and user interactions, making them a cornerstone in applications like Deep Learning (e.g., neural networks), Computer Vision (e.g., image recognition), Natural Language Processing (e.g., language translation), and Recommendation Systems (e.g., personalized suggestions). To leverage matrices effectively, AI relies on key mathematical concepts like Matrix Factorization (for dimension reduction), Eigendecomposition (for stability analysis), Orthogonality (for efficient transformations), and Sparse Matrices (for optimized computation).
The Applications of Matrices - What I wish my teachers told me way earlier (Zach Star, October 2019)
youtube
Transformers are a type of neural network architecture introduced in 2017 by Vaswani et al. in the paper âAttention Is All You Needâ. They revolutionized the field of NLP by outperforming traditional recurrent neural network (RNN) and convolutional neural network (CNN) architectures in sequence-to-sequence tasks. The primary innovation of transformers is the self-attention mechanism, which allows the model to weigh the importance of different words in the input data irrespective of their positions in the sentence. This is particularly useful for capturing long-range dependencies in text, which was a challenge for RNNs due to vanishing gradients. Transformers have become the standard for machine translation tasks, offering state-of-the-art results in translating between languages. They are used for both abstractive and extractive summarization, generating concise summaries of long documents. Transformers help in understanding the context of questions and identifying relevant answers from a given text. By analyzing the context and nuances of language, transformers can accurately determine the sentiment behind text. While initially designed for sequential data, variants of transformers (e.g., Vision Transformers, ViT) have been successfully applied to image recognition tasks, treating images as sequences of patches. Transformers are used to improve the accuracy of speech-to-text systems by better modeling the sequential nature of audio data. The self-attention mechanism can be beneficial for understanding patterns in time series data, leading to more accurate forecasts.
Attention is all you need (Umar Hamil, May 2023)
youtube
Geometric deep learning is a subfield of deep learning that focuses on the study of geometric structures and their representation in data. This field has gained significant attention in recent years.
Michael Bronstein: Geometric Deep Learning (MLSS KrakĂłw, December 2023)
youtube
Traditional Geometric Deep Learning, while powerful, often relies on the assumption of smooth geometric structures. However, real-world data frequently resides in non-manifold spaces where such assumptions are violated. Topology, with its focus on the preservation of proximity and connectivity, offers a more robust framework for analyzing these complex spaces. The inherent robustness of topological properties against noise further solidifies the rationale for integrating topology into deep learning paradigms.
Cristian Bodnar: Topological Message Passing (Michael Bronstein, August 2022)
youtube
Sunday, November 3, 2024
#machine learning#artificial intelligence#mathematics#computer science#deep learning#neural networks#algorithms#data science#statistics#programming#interview#ai assisted writing#machine art#Youtube#lecture
4 notes
¡
View notes
Text
Language Models and AI Safety: Still Worrying
Previously, I have explained how modern "AI" research has painted itself into a corner, inventing the science fiction rogue AI scenario where a system is smarter than its guardrails, but can easily outwitted by humans.
Two recent examples have confirmed my hunch about AI safety of generative AI. In one well-circulated case, somebody generated a picture of an "ethnically ambiguous Homer Simpson", and in another, somebody created a picture of "baby, female, hispanic".
These incidents show that generative AI still filters prompts and outputs, instead of A) ensuring the correct behaviour during training/fine-tuning, B) manually generating, re-labelling, or pruning the training data, C) directly modifying the learned weights to affect outputs.
In general, it is not surprising that big corporations like Google and Microsoft and non-profits like OpenAI are prioritising racist language or racial composition of characters in generated images over abuse of LLMs or generative art for nefarious purposes, content farms, spam, captcha solving, or impersonation. Somebody with enough criminal energy to use ChatGPT to automatically impersonate your grandma based on your message history after he hacked the phones of tens of thousands of grandmas will be blamed for his acts. Somebody who unintentionally generates a racist picture based on an ambiguous prompt will blame the developers of the software if he's offended. Scammers could have enough money and incentives to run the models on their own machine anyway, where corporations have little recourse.
There is precedent for this. Word2vec, published in 2013, was called a "sexist algorithm" in attention-grabbing headlines, even though the bodies of such articles usually conceded that the word2vec embedding just reproduced patterns inherent in the training data: Obviously word2vec does not have any built-in gender biases, it just departs from the dictionary definitions of words like "doctor" and "nurse" and learns gendered connotations because in the training corpus doctors are more often men, and nurses are more often women. Now even that last explanation is oversimplified. The difference between "man" and "woman" is not quite the same as the difference between "male" and "female", or between "doctor" and "nurse". In the English language, "man" can mean "male person" or "human person", and "nurse" can mean "feeding a baby milk from your breast" or a kind of skilled health care worker who works under the direction and supervision of a licensed physician. Arguably, the word2vec algorithm picked up on properties of the word "nurse" that are part of the meaning of the word (at least one meaning, according tot he dictionary), not properties that are contingent on our sexist world.
I don't want to come down against "political correctness" here. I think it's good if ChatGPT doesn't tell a girl that girls can't be doctors. You have to understand that not accidentally saying something sexist or racist is a big deal, or at least Google, Facebook, Microsoft, and OpenAI all think so. OpenAI are responding to a huge incentive when they add snippets like "ethnically ambiguous" to DALL-E 3 prompts.
If this is so important, why are they re-writing prompts, then? Why are they not doing A, B, or C? Back in the days of word2vec, there was a simple but effective solution to automatically identify gendered components in the learned embedding, and zero out the difference. It's so simple you'll probably kick yourself reading it because you could have published that paper yourself without understanding how word2vec works.
I can only conclude from the behaviour of systems like DALL-E 3 that they are either using simple prompt re-writing (or a more sophisticated approach that behaves just as prompt rewriting would, and performs as badly) because prompt re-writing is the best thing they can come up with. Transformers are complex, and inscrutable. You can't just reach in there, isolate a concept like "human person", and rebalance the composition.
The bitter lesson tells us that big amorphous approaches to AI perform better and scale better than manually written expert systems, ontologies, or description logics. More unsupervised data beats less but carefully labelled data. Even when the developers of these systems have a big incentive not to reproduce a certain pattern from the data, they can't fix such a problem at the root. Their solution is instead to use a simple natural language processing system, a dumb system they can understand, and wrap it around the smart but inscrutable transformer-based language model and image generator.
What does that mean for "sleeper agent AI"? You can't really trust a model that somebody else has trained, but can you even trust a model you have trained, if you haven't carefully reviewed all the input data? Even OpenAI can't trust their own models.
16 notes
¡
View notes
Text
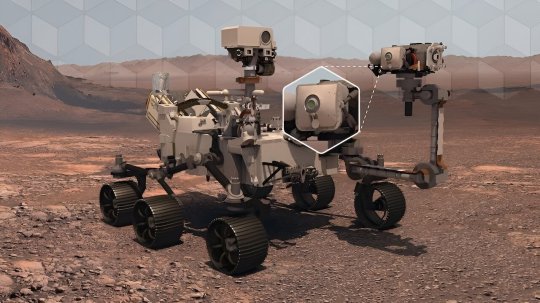
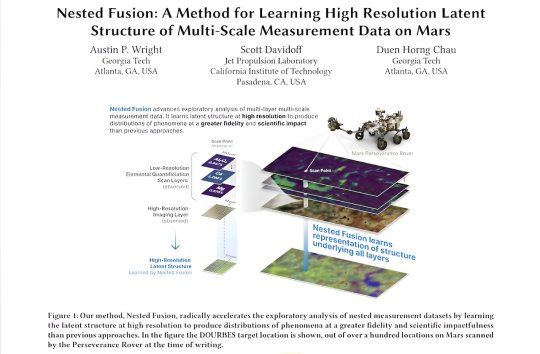
Algorithm used on Mars rover helps scientists on Earth see data in a new way
A new algorithm tested on NASA's Perseverance Rover on Mars may lead to better forecasting of hurricanes, wildfires, and other extreme weather events that impact millions globally.
Georgia Tech Ph.D. student Austin P. Wright is first author of a paper that introduces Nested Fusion. The new algorithm improves scientists' ability to search for past signs of life on the Martian surface.
This innovation supports NASA's Mars 2020 mission. In addition, scientists from other fields working with large, overlapping datasets can use Nested Fusion's methods for their studies.
Wright presented Nested Fusion at the 2024 International Conference on Knowledge Discovery and Data Mining (KDD 2024) where it was a runner-up for the best paper award. The work is published in the journal Proceedings of the 30th ACM SIGKDD Conference on Knowledge Discovery and Data Mining.
"Nested Fusion is really useful for researchers in many different domains, not just NASA scientists," said Wright. "The method visualizes complex datasets that can be difficult to get an overall view of during the initial exploratory stages of analysis."
Nested Fusion combines datasets with different resolutions to produce a single, high-resolution visual distribution. Using this method, NASA scientists can more easily analyze multiple datasets from various sources at the same time. This can lead to faster studies of Mars' surface composition to find clues of previous life.
The algorithm demonstrates how data science impacts traditional scientific fields like chemistry, biology, and geology.
Even further, Wright is developing Nested Fusion applications to model shifting climate patterns, plant and animal life, and other concepts in the earth sciences. The same method can combine overlapping datasets from satellite imagery, biomarkers, and climate data.
"Users have extended Nested Fusion and similar algorithms toward earth science contexts, which we have received very positive feedback," said Wright, who studies machine learning (ML) at Georgia Tech.
"Cross-correlational analysis takes a long time to do and is not done in the initial stages of research when patterns appear and form new hypotheses. Nested Fusion enables people to discover these patterns much earlier."
Wright is the data science and ML lead for PIXLISE, the software that NASA JPL scientists use to study data from the Mars Perseverance Rover.
Perseverance uses its Planetary Instrument for X-ray Lithochemistry (PIXL) to collect data on mineral composition of Mars' surface. PIXL's two main tools that accomplish this are its X-ray Fluorescence (XRF) Spectrometer and Multi-Context Camera (MCC).
When PIXL scans a target area, it creates two co-aligned datasets from the components. XRF collects a sample's fine-scale elemental composition. MCC produces images of a sample to gather visual and physical details like size and shape.
A single XRF spectrum corresponds to approximately 100 MCC imaging pixels for every scan point. Each tool's unique resolution makes mapping between overlapping data layers challenging. However, Wright and his collaborators designed Nested Fusion to overcome this hurdle.
In addition to progressing data science, Nested Fusion improves NASA scientists' workflow. Using the method, a single scientist can form an initial estimate of a sample's mineral composition in a matter of hours. Before Nested Fusion, the same task required days of collaboration between teams of experts on each different instrument.
"I think one of the biggest lessons I have taken from this work is that it is valuable to always ground my ML and data science problems in actual, concrete use cases of our collaborators," Wright said.
"I learn from collaborators what parts of data analysis are important to them and the challenges they face. By understanding these issues, we can discover new ways of formalizing and framing problems in data science."
Nested Fusion won runner-up for the best paper in the applied data science track. Hundreds of other papers were presented at the conference's research track, workshops, and tutorials.
Wright's mentors, Scott Davidoff and Polo Chau, co-authored the Nested Fusion paper. Davidoff is a principal research scientist at the NASA Jet Propulsion Laboratory. Chau is a professor at the Georgia Tech School of Computational Science and Engineering (CSE).
"I was extremely happy that this work was recognized with the best paper runner-up award," Wright said. "This kind of applied work can sometimes be hard to find the right academic home, so finding communities that appreciate this work is very encouraging."
3 notes
¡
View notes
Text
My Day Centering Humans and Meeting Min Jin Lee
Written Mon 10/28/24
Today.... was a crazy day. I think so much happened that I just need to recount it all for future me.
Mid September
Literally over a month ago, I filled out a purple default google form with my information indicating interest in being part of the student Q&A for nobody other than one of my favorite authors of all time. How could this precious opportunity just be a first come first serve basis?
Wednesday, Oct 23
Impatient with over a month of silence, I emailed the Korean professor who sent out the email, and got a response to email the actual organizer. The firehose hit me before I got a chance to follow through.
Friday, Oct 25
Then magically on Friday, I got an email telling me I got off the waitlist to be a part of the Q&A on Monday (today).
Monday, Oct 28
9 a.m.
It was so fucking cold today. This morning I walked over to the Central Square bookstore in my new leather jacket (a la @park-haena), crisp 100% cotton white t-shirt and my mom's blue jeans, italian leather boots (2 in heels), beret hat, and leather gloves. Only to see an active film set and sympathetic looks from the set staff that the store was closed. I walked another 20 minutes to the Harvard book store and was told there that She had come in yesterday and bought out her collection. Here, I learned the power of calling ahead. I was late to media studies and I had skipped all last week so I hauled ass to the T to Kendall and booked it to class.
10:15 a.m.
During Media Studies, we were talking about the difference between supervised and unsupervised machine learning. And the last mile of AI/AGI development, and the human's role in teaching the AI how to do their own jobs. Our activity was using the webcam to train an interactive image classification model, a cool gizmo published by Google. Some times I am so saddened by how much computer science I have to think about in the media class I thought would be my respite. Other times, I am grateful to the class for giving me smaller respites of humanities questions in the field I have chosen to claim for myself.
I take a coffee break for longer than necessary, but in my defense, the coffee machine at the banana lounge brewed slowly and would be even slower for others if I took out the pot mid-brew. A freshman boy struck up a conversation with me, perhaps because I did my makeup today. I thought it was brave and very cute of him.
As class ended, I told my prof that the meeting time she suggested was good. She was so kind to write in a response to my previous assignment a small note of encouragement and support to continue pursuing media studies. I took her up on the offer to discuss more opportunities to engage with opportunities on campus!
11:30 a.m.
Boston is so expensive. A coffee and a croissant from Dunkin is as cheap and hearty as you can get near campus. So I got that right after class at the Stratton student center. Small cappuccino with half pump of pumpkin syrup and the ham and cheese croissant stuffer.
I settled in in the third floor wellbeing lounge and did an activity that I was reminded of in class. An AI chatbot, but with your future self, that was done in a research project that my LinkedIn friend worked on. Honestly, the hour I spent on that was actually quite disappointing. Probably because the LLM wasn't big enough, or wasn't trained on enough data, or wasn't fine-tuned with human feedback. But the advice 60-year-old Lucy provided was generic, and she didn't take into consideration the lengthy form responses I composed, totally misreading my personality.
That was sooo much more disappointing than it should've been, because I so deeply desire that connection with an elder and wiser being... but when your grandparents are dead and also so culturally different if they were alive, there are not really many options.
1 p.m.
I saw Her on the way into the room. She was dressed very stylish, and I know that because she was dressed like me, but better. Her leather black boots were unique. They were 4 inch heels but platform but narrow. Her jeans were straight and made her legs look so long. Her suit jacket was slim but not short and didn't try do that shit where it awkwardly peplums at the waist. She was wearing a white dress shirt but under another layer, to combat the cold: a cropped black knit vest or cardigan with a pattern that was neither old or trendy, but classic. Even her big gold hoops, were not circular and basic, but drooped elegantly. I didn't expect her to be so pretty.
I was early and so I walked in and claimed front center, surprised to see that the best two seats were taken by two girls I already knew over the past three years. They were nice enough to respond when I asked them questions, but it was clear that they were friends and Not Interested in being friends (with me). It was fine. Thinking about what I wanted to ask Her about Pachinko and Free Food For Millionaires, I was pre-occupied and basically shaking in my boots, so I texted Hanu for help. She responded immediately, which is rare, but in that moment I forgave her for every time she had not responded to me, because I actually really needed her then and I think she could sense that.
<the Q&A itself>
Her biggest advice was to talk to your peers. Which I thought was funny, because I tried, and LOL it was just ok.
She said many things that i expected. Geography means a lot to a person.
She said some things that i learned. To take away the sheer privilege of being at MIT. And to be Korean and do many things. Fill many roles in society. Where is the female Korean pilot?
She said some things that i was shaken by. Casey was not necessarily surrounded by bad men. Her first white boyfriend cheated with two sorority girls, but was that a sign he was a bad person? Or is that an innately human action? I don't understand the distinction.
She said she too was not always ready to be the best partner. She said as a writer, you should give each of your characters some patience and love. Hansu, might be her excetpion.
<the Keynote Talk>
I walked in and saw two girls I knew but were never that warm to me. I said hi, and we were cordial, and I said I"ll just sit behind you two. Rather just focus on the speaker today. And I scooted past an elder asian woman with the tiniest frame ever.
Then all the big wigs started mixing around in the front. Sally. Deans. Nobel laureates. Then one of my favorite professors walked in, and stopped by my row.
"do you know each other? I know you both from very different places." One influential talk later, I had lunch plans for Saturday with the korean lady next to me. And future plans for coffee with my professor.
Is it okay to ask a professor to pick their brains? Is it offensive?
Love people. Protect your peace. And walk down the path you actively choose.
3 notes
¡
View notes
Text
[A MewTube video from Team Curiosity's official channel is attached. The title is "TCBFSCâDr. Ryan Alston: Universe TypesâParallel vs. Alternate
Transcript:
Dr. Ryan Alston walks out from stage left. He approaches the podium without looking at the stage or behind himself, keeping his eyes dead focused ahead. As he sets a small deck of flash cards in front of him and switches the mic on, he leers out over the crowd. With the PowerPoint behind him flipped on, he leaned into the mic and spoke in a stern, yet stilted tone.
Ryan: Good evening. I am Dr. Ryan Alston, admin at Team Curiosity. I'm a theoreâphysicist. I am a physicist specializing in space-time anomalies. Today, you're going to learn about the multiverse. Specifically, the difference between parallel and alternate universes.
He seems to have the wind taken out of his ass momentarily at the flubbed line, but maintains speed and flips the presentation slide. The next slide simply says "timelines" and has an illustration with a person standing at the base of a branching path.
Ryan: Now, to clarify first: I'm not talking about timelines. Timelines can create something akin to a parallel universe effect, but time itself being relative, it is entirely possible to shift forward, backward, and along different branches of a timeline within the same universe. There are a few pokemon who display this traitârare as they are.
He turns to the next slide, gesturing to it with his open hand. It simply says "parallel universes."
Ryan: I am talking about universes. Parallel universes are the ones most often confused for different timelines, and are fairly well-documented by Alolan scientists. These universes can appear wildly different than your own, even containing different creatures. However, the same fundamental physics and base concepts will still apply. The Ultra Beasts of the wormholes in Alola are simply pokemon from another universe, and they follow the same rules as powerful pokemon when brought here.
He flips the slide. Silhouettes of Solgaleo and Lunala are portrayed.
Ryan: There are a few pokemon known to be able to traverse the universes. They're... Again, rare. But they are able to traverse to parallel universes and return safely. The research is secretive, and we are working on uncovering the true extent of their capabilities, but it is there.
Ryan then flips to the next slide. It says "alternate universes".
Ryan: Alternate universes are entirely different. They abide by different physics, different rules. The fundamentals have shifted entirely. Maybe they look vastly different, maybe they don't, but their core set of universal constants do not align with your own. As some of you may know. I am a faller. I am from one of these universe types. Pokemon do not exist, and our laws of physics vary drastically. This makes us an alternate.
He flips to the next slide. It is blank. His expression almost seems solemn, but his brow remains stern.
Ryan: ... As far as we know, there are no ways to force travel between these universes. There are known cases of people falling into this universe from an alternate, but there are no cases of them being able to return. Research is in progress to change this, but it's all experimental now.
He pauses for a moment, then shuts the presentation off. He momentarily shuts the microphone off to take a breath before flipping it back on.
Ryan: We may track down a pokemon at some point in the future capable of travel, or maybe we might build a machine to open the path by force. Either way, it is something we cannot accomplish so long as the research currently in place remains as secretive as it is. Science is built on the shoulders of giants, after all. However, when it comes to raw data, that's for the scientists to figure out the intricacies of. As for the rest of you... I hope you at least remember this difference.
He took a bow, then walks off of the stage as stoic as he came in.
23 notes
¡
View notes